Now that we’ve covered tracking phage metadata and building identities for our phages, it’s time to expand our focus. We should now consider how our phages fit into the rest of the collection of biological assets in our biobank. How do we “accession” our phages into this biobank, and what should we take into account? How do our phages relate to our other assets, like bacteria and antibiotics?
In this third post in the informal and conversational “Phage Data series”, I’ll step through how I think about building a biobank data system.
Building up a library
We often refer our collections of biological assets as “bio banks”, but the “bank” analogy doesn’t completely fit. We don’t invest or collateralize our assets, and we often don’t charge fees to manage others’ assets. Instead, our “bio banks” are more like “bio libraries” — we add new items to our libraries, we maintain and grow the number of copies we have of each item, and we maintain, borrow, lend, check out, and check in our items much like a library does.
In terms of book libraries: what’s in a book, and what’s being collected? A book has core identifying characteristics like title, author, a unique identifier (ISBN), and the text within the book itself. Libraries don’t actually collect the core identifying characteristics per se — they collect copies of those characteristics. Each copy, bound in the form of a book, is assumed to be an exact copy of those core identifiers. Additionally, each book has its own set of metadata: when was it checked out to whom? When and who checked it back in? What’s the condition of the book? (If the last page has been ripped out… is it still a valid “copy of the core identifiers”, or does it need to be repaired or destroyed?) Have more copies been ordered? At any moment, book A of Arrowsmith could be on the shelf, while book B is checked out.
What is “accessioning” and how do you do it?
When we add a new book to our book library, we record its core identifiers. If we just added Arrowsmith to our library, we’d add the title, its author Sinclair Lewis, and maybe a description). We separately accession the copies of the book.
“Accessioning” is just a fancy word for “adding” an item to a library. When we add a new copy of an item to our library, each copy needs to have a unique identifier (“accession ID”) to those items (e.g. Arrowsmith_A, Arrowsmith_B, …). This allows us to track the details and location for each copy of the book.
We might also want some information that’s not tied to the identity of Arrowsmith, or each copy of the book. For example, do our copies of Arrowsmith go in the Medical Mysteries, Pulitzer Winners, or Science Fiction section of the library? These are subjective choices that could vary between libraries — one might put it in the Science Fiction section, while another might put it in the Pulitzer section. These subjective descriptors of Arrowsmith need to be recorded, but should be kept separate from the core identifying and instance data.
Data type |
Examples |
Core Identifying Data |
Book name, author, ISBN |
Descriptive Data |
Science fiction shelf; “top picks” category; “should be made into a movie!”; reader feedback and ratings; popularity of book over time |
Instance Data |
Accession IDs; book_A: on shelf; book_B: checked out |
Building a library of biology assets
We can treat our “assets” in our bio bank — like phages, bacteria and plasmids — like books in our previous example. We store “instances” of phages and bacteria in our bank, similarly to how libraries store copies of books in a library.
We would store data about our bio assets in the same way as well. We would need to know each of our items’ core identifying data, like a phage’s name, isolation host, and GenBank accession ID. We would also need to know descriptive data, like “who’s managing these phages”, “who sent them to us” and “what MTAs are attached to them”. Lastly, we would also have instance data, like “where are the vials stored for phage A” and “are any experiments currently running for bacteria B”.
This analogy extends past phages and into every asset in the biobank, just like the book library analogy extends past just books as well. A library might extend the book analogy into DVDs, music records, and even gardening and electronics kits. Similarly, we can use the same model to inventory our phages, bacteria, plasmids, and host range test panels.
Keeping track of our instances
Just like a library needs to track what happens to individual copies of each book, we need to track each “copy” of our bio assets in our bio library.
I’m using the word “instance” to describe a “copy” of a phage. If we put a phage into four vials in a freezer and one vial in the refrigerator, our library would have five instances. I refrain from using words like “sample”, “specimen”, or “isolate” to describe copies in a library since these can have very specific meanings, which quickly result in confusion. Our library can have five instances of an environmental specimen, five instances of a bacterial isolate, and so on.
Treating our biobank as a library of instances helps us reason about certain scenarios. For example, we might discover that one phage turns out to be two different phages. We would isolate the two phages, characterize them, and confirm our suspicions. How do we record this information? Firstly, any characterization done on the original phage was actually performed on the cocktail/combination of our two phages. We create two new phage entries for our two new phages, and record these new isolates as new instances in our system. The vials of the initial phage (that turned out to be two phages) are now instances of a phage cocktail! Any vials can just be treated as a combination of two vials. Any characterization data can just be reclassified as the combined effects of two phages.
Using the instance analogy also helps with other areas like licensing and Material Transfer Agreements (MTA). Instances help us track what’s been done with each phage, and what we’re allowed to do. For example, an MTA might state that we are not allowed to replicate or distribute a phage (in licensing, this is like saying you are not allowed to make copies of a DVD and share them with friends. Some of you might remember the “FBI” warning screens of old DVDs!). If our system treats every copy we make of a phage as a new “instance”, this helps us know if we’re upholding our end of an MTA. A system like this helps with tracking the provenance (where something came from) of bio assets.
Similarly, when we ship/receive vials of phages among collaborators, we can attach our own MTAs to those instances. If a collaborator publishes on that instance, we can connect those publications with that instance. If we (or a collaborator) makes duplicates or derivatives, we can have those instances inherit the licenses of the original instances (this is how some software licenses work). If that instance turns out to actually be a combination of two phages, we can now trace and inform anyone who’s used and published on the phage. Equally, if we characterize the phage further, we can update anyone who’s ever received that instance of the new characterization information.
Keeping track of what we know about our instances
Once we have our core identifying characteristics defined for a phage, what other information do we need to collect?
Remember that books have both descriptive and instance data. Descriptive data is like “which section does this book belong in” and “what do readers think about this book”. Instance data records things like “this book is in bad shape” or “that book is checked out”. What does this look like for phages?
Descriptive Phage Data |
Instance Phage Data |
Who is responsible for it? (main contact, lab manager) |
Where is it? Location, box, etc. |
What to do when person in charge leaves? |
Is it “expired” / when does it need to be re-checked and amplified? |
Methods, handling, SOPs, “onboarding” documents |
Actual storage, shipping conditions |
MTAs and licenses (what can I do with it) |
Who has it been sent to? |
Nagoya (what paperwork do I need to share it) |
Batch-level certification (Sciensano Phage Passport) |
Who / where did this phage come from? |
Work performed on each instance |
Where is it published |
Events occurred on the instance (e.g refrigerator failed) |
Where is the data? (TEM, spot assays, sequence) |
|
Who is allowed to work with it? |
|
What ongoing projects use it? |
|
Preferred conditions for storage, shipping, etc. |
|
Where is it published? |
|
Data collected for this phage, from all instances |
|
Most information we’ll know about a phage, like host range, plaque morphology, propagation, and antibiotic synergy aren’t core to the phage itself. Previously, I called these “conditional” or “mutable” characteristics. These are characteristics that depend on the environment (e.g. you can’t have plaques if there’s no bacterial isolate present). Most of this information will come from data collected from various processes, assays, and events **that happen to instances of your bio assets.
With this asset-assay relationship in mind, how do we collect and store conditional characteristics around our phages, bacteria, and other bio assets, from relevant events and assays?
Keeping track of processes, assays, and events
We can define everything that happens to our instances as events and actions. We can further break down actions as processes and assays.
In our book library example, events could include check outs and returns, and actions could be “repaired a book”. Each event has some information around it, like what copy was checked out, and who/when was it checked out.
For our bio library, we can similarly track events and actions. The main difference is that our actions and events will generate new data that are added to the core and descriptive characteristics.
Events are passive occurrences that happen to our instances. For example: the sample arrived via DHL; the freezer broke and instances need to be re-checked, etc.
Processes are intentional actions we perform on our instances. For example: we isolated phage X; we created five instances of it in these vials, and they’re each at X PFU/mL and stored in this box/drawer/freezer; we shipped one instance out to a collaborator, and here is the shipping manifest. This data we collect should be descriptive of our instances, and not core or conditional characteristics of our phages and assets.
Assays are intentional actions we perform to gather data and insights. For example: we did a spot assay and know the phage infects these bacterial isolates; this phage is able to lyse under these conditions but not those conditions, etc. This information is core or conditional, and should apply to every one of our phage/asset instances.
We can represent anything we know about our phages (and bio assets in general) either in absolute terms or in terms relative to the assays and experiments we’ve performed. We could state, in absolute terms: “Phage A’s host range includes isolates X, Y, Z”. We could also state a host range in terms of data collected from assays: “Assay 1, 2, 3 shows Phage A clearly plaque on X, Y, Z, but not on D, E, F”.
Tracking data recorded from assays (along with conditions like growth conditions) helps us establish the range of information we’ve collected around a phage. We can say that the host range is X, Y, Z based on assays 1, 2, 3, but also that the host range is not D, E, F. The lack of spot assay data on bacterial hosts G, H, I and J merely indicate we haven’t done those assays, not that those aren’t within the phage’s host range.
The body of knowledge we have on a phage is then the sum of the data collected on the phage or asset. By defining a host range as data collected from assays, we get a more complete picture of what works, what didn’t work, and what has and hasn’t been tried. This would lead us to know whether a host range is based on the evidence of no plaques, or merely the lack of plaque data.
Below is an idea of how processes, assays, and event types can be separated. This is by no means an exhaustive list. It’s also not final — this is just how we are currently approaching how to think about data collection at the moment.
Types of Processes actions pertaining to a subset of instances |
Types of Assays actions that gather insights to core characteristics |
Types of Events passive occurrences to instances |
Isolation |
TEM / Imaging |
Shipping + receiving events of instances |
Purification |
Genome sequencing |
Freezer broke; instances need re-checking |
Propagation |
Genome annotation |
Phages possibly “expired” and need to be re-checked |
Storage |
Host range spot testing |
|
Shipping |
Host range kinetics |
|
Manufacturing |
AMR synergy kinetics |
|
Centrifugation |
|
|
Filtration |
|
|
MTA documents shared and signed |
|
|
Destruction/consumption |
|
|
Request |
|
|
Sample collection |
|
|
Accessioning |
|
|
Creation of derivative (evolution/training/engineering) |
|
|
PFU Count |
|
|
Publication |
|
|
QC & Certification |
|
|
DNA extraction |
|
|
Are we overthinking it?
Before we continue further, we should stop and check ourselves.
Are we doing more than we need to? Do we even need to “accession” phages?
If we worked on a few dozen phages and bacterial isolates, we could probably stick to using Excel for everything. We should definitely NOT create solutions in search of a problem!
Excel is a fine tool for tracking information like bio-banking samples. If you have a small, synchronous (everyone on the same time zone and in the lab at the same time), tight-knit lab that communicates and works well, and documents processes and data in a way that the entire team can see, you probably could stick to Excel, Google Sheets, or even direct messaging. Writing a slack message or sending a quick message would be much faster than updating a complex, full-featured database.
If you’re anticipating hundreds of samples, asynchronous work (people working at different times), and new lab members who need to be quickly caught up to speed, then putting the work up front in a proper documentation and data system should save you more headaches later.
If you’re like us, you probably don’t have data stored relative to processes and assays yet. You probably want to progressively convert your data and practices into a new system like this, without having to invalidate your old data.
How to put these ideas into practice
Unfortunately, neither Excel nor Google Sheets are very good at putting these ideas into practice. Spreadsheets are great for laying out two-dimensional data. However, they fall apart when connecting multi-dimensional pieces of data. The problem is that spreadsheets aren’t relational.
In a spreadsheet, listing out species a phage infects is easy: you just type out the strains. However, you can’t derive a phage’s host range from the results of assays between a phage and a library of bacterial isolates. This is a relation between a phage, an assay, and isolates. The host range is an outcome of the assay, rather than a property of the phage or the isolate. When we record the data, we only record the spot test itself, the phage and bacterial isolate used, and the results. The results could then be rolled up back into both the phage and isolate records — “PhageA spots on IsolateA, from SpotTest1”, and similarly “IsolateA is susceptible to PhageA from SpotTest1”
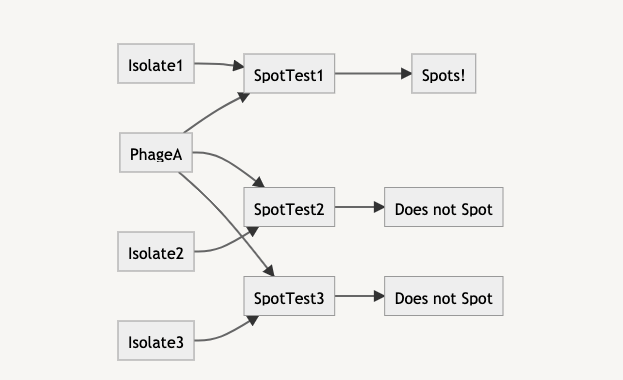
The benefit of portraying data like a relationship is that outcomes are now the results of assays, rather than “absolute” values of a phage. By entering the outcomes of each spot assay, we would indirectly know the host range of the phage. This data model helps us answer the question: is this the phage’s host range because it didn’t plaque on the isolates, or because the spot assay hasn’t been done yet?
Tracking relationships like this in a spreadsheet is a lot of manual work, and I wouldn’t recommend it.
Luckily, there are systems out there — from spreadsheet-like relational tools, to tools that let us connect Google Sheets and convert spreadsheets and CSVs to more relational data. These tools let us start in spreadsheets (where our data already is), and progressively move into more bespoke bio banking interfaces.
Looking Ahead:
In the previous issues we’ve touched on the concepts of keeping track of phage metadata, and naming a phage / building an identity for a phage. In this issue we touch on tracking the relationships between phages and phage hosts, the work performed on them, and recording the outcomes.
In the next issue, we’ll explore various tools for bio banking, from using VLOOKUP in Excel and Google Sheets, to using no-code relational database tools. We’ll also touch on database design and building your own database and app from scratch… and how to combine the best of both worlds. Stay tuned!
Extra special thanks to Ben Temperton from the Citizen Phage Library for discussing and figuring out databases and schemas with me!
Special thanks to Jessica Sacher, Evelien Adriaenssens, and the Phage Australia team (Ruby Lin, Nouri Ben Zakour, Stephanie Lynch, Jon Iredell) for helping me hash some of these ideas out.
More special thanks to various phage labs and biobanks we’ve spoken to over the years about data management. Some of these labs include: Queen Astrid Military Hospital, Sciensano, the Félix d’Hérelle Reference Center for Bacterial Viruses, DSMZ, ATCC, NCTC, TAILOR, Israeli Phage Bank, The Bacteriophage Bank of Korea, Fagenbank, Japan Phage Bank, and many more, throughout the years. Thanks so much for putting up with my incessant questioning!
Also thanks to Stephanie Lynch and Atif Khan for all their help with news, jobs and community sections this week!