When working with phages, we often find ourselves performing the time-intensive monotonous task of counting plaques on Petri dishes for a variety of assay types. To help solve this problem I developed OnePetri, a set of publicly available machine learning models and a mobile phone application (currently iOS-only, Android version under development) that accelerates common microbiological Petri dish assays using AI.
A task that once took microbiologists several minutes to do per Petri dish (adds up quick if you have many plates to count!) could now be mostly automated thanks to computer vision, and completed in a matter of seconds…don’t blink or you’ll miss it!
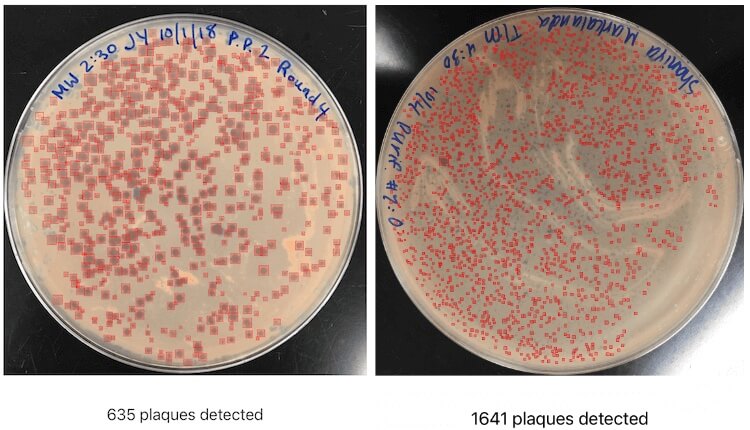
OnePetri screenshot showing Petri dishes with hundreds and thousands of plaques detected, all within a matter of seconds.
Teaching Computers to “See”
Artificial intelligence, computer vision, and machine learning are all really trendy marketing buzzwords, but also represent key milestones in technology which allow for an entire new domain of research, analysis, and applications to be realized.
IBM defines computer vision as a field in artificial intelligence which allows computers to derive useful information from images or videos, meaning that computers can be taught to “see” and interpret images the same way humans can. In fact, computer vision is what the photos app on your smartphone uses to let you search for an image by its contents - searching for “cake” would bring up all your photos of phage cakes, for example!
Teaching a computer to see is no small feat, however, and significant research and development has been done throughout the years to make this process a much simpler one than it once was. Real-time object detection models, such as the YOLO family (YOLO stands for “You Only Look Once”), often require significant amounts of training data containing the object(s) of interest for the models to be trained successfully and be robust enough under a wide variety of conditions. There are several pre-trained models available for public use, however I couldn’t find which were already trained to detect common lab objects like Petri dishes, never mind phage plaques. This meant that I’d need to start off by training models of my own for this purpose specifically.
Under the hood, OnePetri sports two object detection models (YOLOv5s) for Petri dish and plaque identification. These models were trained on a diverse set of images from the Howard Hughes Medical Institute’s SEA-PHAGES program. I’m extremely grateful for their contribution of over 10,000 plaque assay images to this project, as this wouldn’t have been possible without their support!
A total of 43 images were included in the initial dataset for OnePetri’s plaque detection model with the following split: 29 training, 9 validation, 5 test. Although the number of images is quite small, each has a large number of plaques (~100 plaques per image on average), giving the algorithm plenty of data to train on. To increase the training dataset even further, I also applied tiling, hue shift, and grayscale augmentations to accommodate different colours of agar and lighting conditions. The Petri dish detection model was trained on 75 images, with an additional 23 in the validation group. The models were trained locally using an NVIDIA RTX 2000-series GPU.
Precision and recall are two commonly used metrics in machine learning used to benchmark new models, comparing the results returned by AI to the same data which has been analyzed manually. Precision measures the portion of detections in a dataset that were actually correct, determined by taking the number of correct plaques detected and dividing by the total number of plaques detected. Similarly, recall measures the proportion of real plaques that were identified correctly, taking the number of true plaques detected and dividing by the total number of plaques which should have been identified. For both metrics, higher values are better and represent models which return more relevant results than irrelevant ones. After 500 cycles of training, the plaque detection model had a precision of 93.6% and recall of 85.6%, while the Petri dish model had a precision of 96% and recall of 100%. With these trained models in hand, I was now able to move on to creating a mobile app wrapper around the trained models.
From Idea to Reality: Bringing OnePetri to the iOS App Store
Once trained, the models were converted to a format that would be compatible with Apple’s CoreML framework, and so began the process of building OnePetri for iOS! After only a few weeks of Swift coding, I had a functional prototype which was put through its paces by a dedicated group of beta testers (thanks to all those who tested OnePetri early on!). The OnePetri Swift source code is now publicly available on GitHub under a GPL-3.0 license. The trained models are also publicly available on GitHub. OnePetri is also available for download worldwide (for free!) on the Apple App Store.
When using OnePetri, the user is first prompted to select or take a photo for analysis. This image can contain any number of Petri dishes for analysis, though for small plaques typically 1-2 plates per image is recommended to maximize the number of pixels per plaque. The cropped Petri dish of interest is divided into overlapping 416px x 416px squares (tiles), and plaques are inferred serially on each tile. After removing duplicate plaques, which can occur due to the use of overlapping tiles, the final plaque count is shown and bounding boxes are drawn around each plaque. The user can also use the plaque assay feature to automatically calculate phage titre based on plaque counts from tenfold serial dilutions.
All image processing and inference is done locally on-device, meaning OnePetri can be used even without internet access once the app has been installed, and that all images analyzed remain private. Regular feature and model updates will require internet access to download.
The Road Ahead: What’s Next for OnePetri?
Going forward I’ll continue to iterate and improve upon the models included in OnePetri by incorporating user-submitted images, as well as additional images from the HHMI’s SEA-PHAGES program, into the training datasets. In addition to plaque counts, I’d like to add proper support for bacterial colony counting and other assays (though I have heard from users that the current version works quite well on CFUs too, so worth a try!) I’m also working on bringing OnePetri to a wider range of platforms, including a native Android app, increasing access to this time-saving tool to the entire phage community - so stay tuned!
If you have any questions about the computer vision model training process, or OnePetri in general, feel free to reach out by email. I’m looking forward to seeing what the phage and microbiology fields do with computer vision in the years ahead, it’s a really powerful technology which has great potential for its research applications!
Additional resources
OnePetri website: https://onepetri.ai
App Store listing: https://apps.apple.com/ca/app/onepetri/id1576075754
iOS app source code (Swift, GPL-3.0): https://github.com/mshamash/OnePetri
Trained models: https://github.com/mshamash/onepetri-models
Training data/images for plaque detection model (CC BY-NC-SA 4.0): https://universe.roboflow.com/onepetri/onepetri
Many thanks to Atif Khan for finding and summarizing this week’s phage news, jobs and community posts, and to Lizzie Richardson for editing!