Hi everyone,
By now you’ve probably undoubtedly heard of OpenAI, ChatGPT, Large Language Models, and other buzzwords. You’ve probably even tried it a few times yourself!
In this casual post, we’ll briefly touch on how it works, when (and when not) to use it, and some ideas on how you can save time with ChatGPT.
What are LLMs?
I’ll keep this part very condensed, as there are many articles, books, videos, and courses on the subject.
In very broad strokes, ChatGPT and (other similar ones like Bard, Claude or Cohere) fall under the category of LLMs, or “large language models.” These are programs that use predictive models to recognize, predict, and work with text. Basically, when given some text, they’ll use probability to generate more text.
How they generate text, and how accurate that text is, depends on all the text that’s used to create the model (the training data), and how it’s configured to respond to a user (e.g. fine tuning) in a certain way. While ChatGPT is tuned to answer questions in a Q&A format, others could be used to predict molecular phenotypes from DNA sequences.
The process of getting ChatGPT to write exactly what you want it to write takes some guesswork. The stochastic nature of getting language models to do what you want is still kind of “black magic,” so much so that there’s a term for it — prompt engineering. In essence, it means writing clear instructions and spelling out exactly what you need, without any assumptions — kind of like when writing standard operating procedures for the lab.
The stochastic, almost serendipitous nature of language models is both their biggest strength and drawback, and with that in mind, there are lots of things you can and should do with it!
Language models in Nature
Speaking of serendipity, Nature Careers just put out a blog post on how postdocs are using ChatGPT in their daily work. A few surprising details:
- Only 1/3 of respondents use ChatGPT. Maybe more aren’t using it because they don’t find it useful, or because they’re restricted by their institutions?
- Engineers make up almost half (44%) of the respondents who use ChatGPT (surprise!). What’s surprising is that social science and biomed follows closely behind
- Most popular use cases: refining text (surprisingly, I don’t do this; I have Jess to do this for me…), generating + editing code (I do this), finding literature (do NOT do this; I’ll go into why later)
Some cool use cases from the article:
- Using ChatGPT to write formal Japanese emails, to overcome language barriers and more effectively communicate with other lab members. Tip: ask ChatGPT to “translate (this text) to Japanese)”
- Using ChatGPT to summarize articles and papers. Tip: ask ChatGPT to “explain (copy and paste a paper) like I’m 5”
- Explaining code. Tip: ask ChatGPT to “explain (this code)” or “rewrite (this code) from python to javascript”
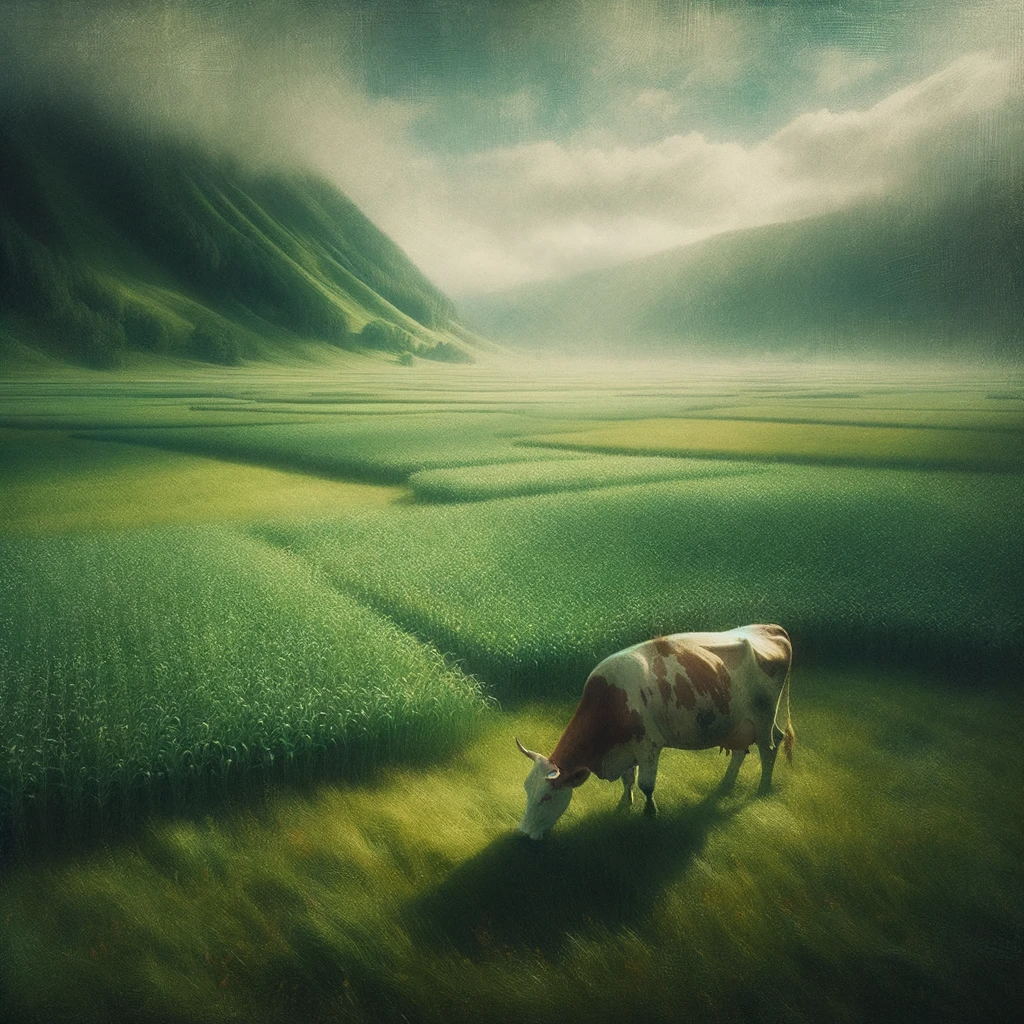
Fig 1. A cow in a field, generated by ChatGPT because I can.
What AI models can and can’t do
It’s very important to remember that language models are kind of like weather predictions. They’re really useful, but are wrong all the time — because they’re statistical machines. Language models can’t reason or do math, but they can pretend to do math based on the math examples they’ve seen before. They can also pretend to write confidently while making stuff up — this is called hallucinating.
As long as you pretend it’s an over-confident first-year student, you should be ok.
Do’s with ChatGPT:
- Use for idea generation. Tip: I walked for an hour in the park, having a conversation with GPT-4’s voice mode, to get ideas for this article. It’s great to bounce off ideas with, and can quickly get you over your writer’s block.
- Cross Examination & Fixing Errors: Have it quiz you, if you’re prepping for an exam. Have it look at your grant proposals or experimental designs or standard operating procedures. You’ll be shocked at how good it is at coming up with ideas that never crossed your mind.
- “SWOT” analysis; counter-arguments: Have it tear down your presentations and other work, before your PI or lab meeting does.
- Use it casually, to create drafts, edits, variations: I often use ChatGPT to help me come up with variations and new directions.
- Spelling & Grammar: Although its bad at generating less-than-cringeworthy writing, it’s good as a grammar check.
- Use it to summarize articles and explain papers: We’re all short on time. Use ChatGPT to give the gist of an article or paper before you decide to spend 30 minutes reading it.
- Translations: for blog posts, or to communicate with others in their native language. My parents use ChatGPT to read English and other articles. Soon Youtube and Spotify will have a way to listen to any podcasts in any language.
- Use with oversight: Just like with first-year students: always double-check what it wrote!
- Use it with Code: Use it to convert code from one language to other, or to even write code from scratch. (GPT-4 is really good at this. You’ll still need to read through it to watch out for obscure bugs though; you can even ask it to “identify bugs and race conditions” after it’s generated some code, and it’s pretty good at fixing things).
- Generate excel formulas: Give it a couple of rows of data, and ask it to generate a formula to do XYZ. Or you could just have it generate the data. 99.9% it’s 100% correct… so take that with a grain of salt.
- Generate Mermaid charts / visualizations: GPT-4 is very good with anything code-based, including graphing libraries like MermaidJS or D3js, or libraries in Python or R. Just give it some data and ask it to visualize it. It’s kind of magical.
- Explain Images: GPT-4Vision is very good at explaining graphs, obscure microbiology research paper figures (ahem), and even extracting data from screenshots of tables into markdown and readable CSV data! The bad part is that it sometimes, though rarely, makes mistakes (though probably at a rate lower than I make mistakes).
- Generate Images like blog post covers (like this one!)
- Get a Vibe Check: Use it for getting ideas, and vibes. Use it to guide you in the directions you need to go, but you have to do the rest of the work yourself. It can set you down a path, but you have to walk it yourself… that’s what makes your work yours!
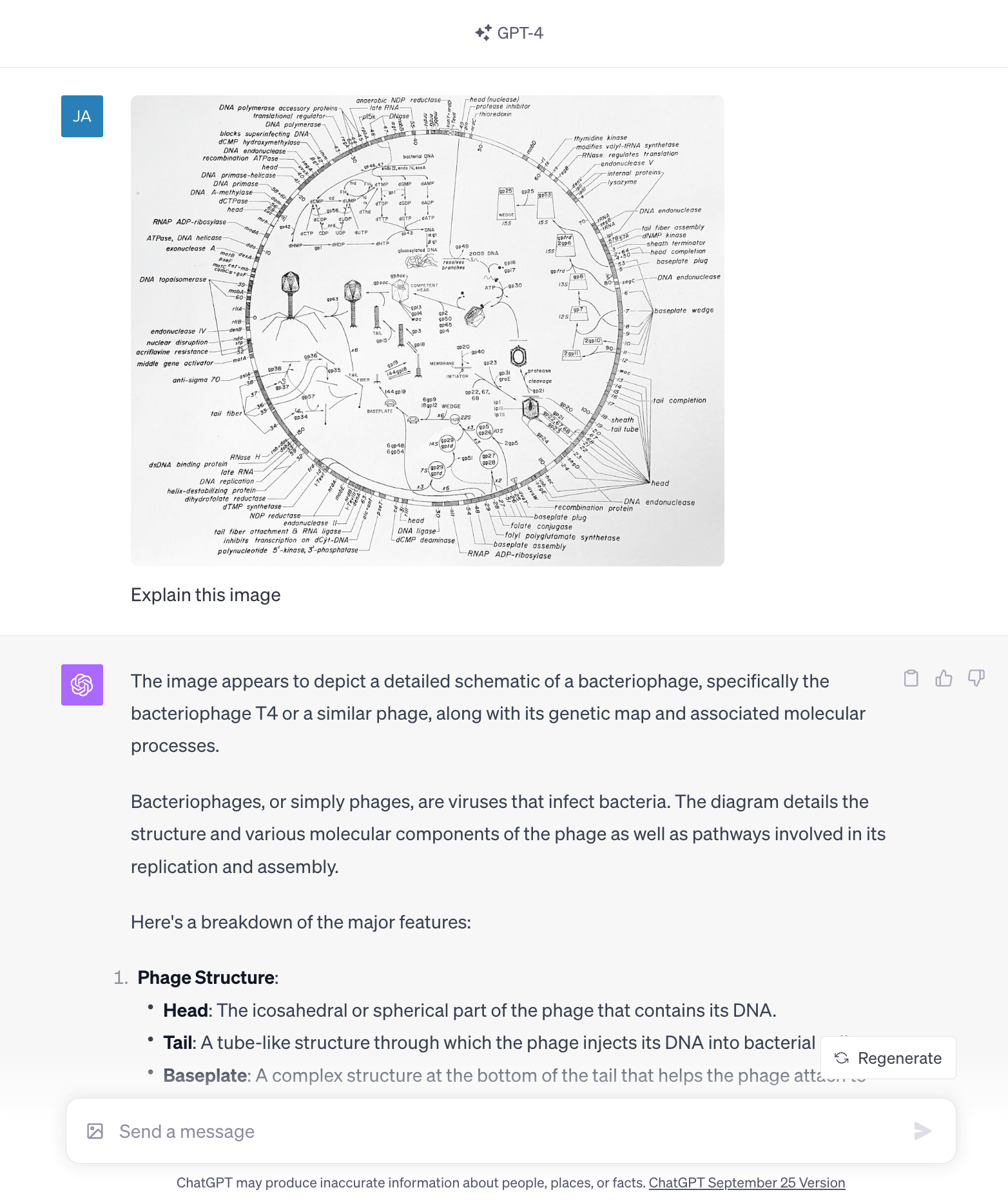
Fig 2. GPT-4 explaining the diagram without any prior knowledge. It tries to go into the full genome map later on but gives up — you’d have to reprompt it, but it’s still impressive.
Don’ts with ChatGPT:
- Don’t put any private data in ChatGPT: Don’t put your address or credit card or other important information. The conversations are monitored by staff, and can even be used to train future iterations. This means that your credit card could accidentally show up in other people’s chats! If you really need to, you can use its “incognito” mode.
- Don’t write articles or emails with it: Avoid using it to write blog posts, job posts, or emails; it sounds robotic and cringeworthy. At best it makes you sound like a robot; at worst, it’ll make up incorrect stuff and make you look stupid. What was previously thought of as professional writing styles are now easily mimicked with language models. This means that your writing needs to have character, and needs to stand out from the background noise.
- Don’t use it as a Search Tool. Don’t use it for Facts, or when Accuracy matters! It doesn’t know everything on the internet, and it tends to get citations wrong. If you feed it papers (by copy/pasting them), it’ll cite them correctly, and some apps like Bing Chat and GPT-4 can browse the internet, which means it’ll get the right answers. But much of the time, instead of saying “I don’t know” it’ll gladly make something up.
- Don’t use it for math or reasoning. It can trick you into thinking it’s doing the math, but it’s mimicking the data its trained on. One way around this is to use GPT-4’s Data Analysis (formerly Code Interpreter) plugin, which writes code to analyze data.
- Don’t use it to generate high stakes, important things. It loves to make stuff up!!!
Real-life use cases
Here are some real-life use cases from Jessica:
- Using chatGPT to make buffers: Making buffers always causes me unnecessary grief in the lab, so I’ve started using ChatGPT. I gave it a list of buffers and contents (e.g. Regeneration buffer: 20 mM HEPES, 1 M NaCl, 2 mM EDTA, pH 7.5), and asked it to make me a step by step protocol for making 500 mL. I asked for a list of materials needed (incl. glassware, chemicals, ph meter, weigh boat, etc). I asked it to format everything into a table I could paste into Excel, that listed all the required ingredients, their molecular weights, and calculations for mass and concentration. It accurately came up with molar weights and calculated most things properly! Also, I asked it to do several recipes at a time, ie. all the buffers I needed for the day. That way I could assemble one cumulative set of ingredients and materials, and do it all together. (Note that I used the paid version, GPT-4).
- I’ve used it to improve my CV and write resumes, ie. asking it to format some work I’ve done into a resume section (e.g. give it an abstract from my thesis, ask for it to write the section on the skills I developed/accomplishments during my PhD).
- I’ve used it to pull numbers from a PDF quote from a company into a spreadsheet so I can compare multiple pieces of equipment.
- I’ve used it to turn a conference abstract into an outline for a presentation given the length, and to allocate times and number of slides accordingly.
- I’ve asked it to explain hazards of chemicals (”why is it dangerous if bleach and ammonia are combined? what should I do to dispose of 1M NaOH?”).
- I’ve asked for ideas on how to filter a phage lysate that isn’t filtering.
- I want to start using it to plan lab experiments given what I want to do in a given week, seeing if it can come up with which to start when, what to do during breaks of an experiment, etc. (This last one is inspired by Jan using it this way for cooking…)
Jan’s / Editor’s note on the last one: I use ChatGPT to plan cooking for me. I’ll give it the vegetables and meats I want to roast in the oven, and it’ll give me a table of when to add the next ingredient on the pan — this way everything comes out perfectly caramelized. I’ve done this four times now, and every time the food has come out absolutely perfect.
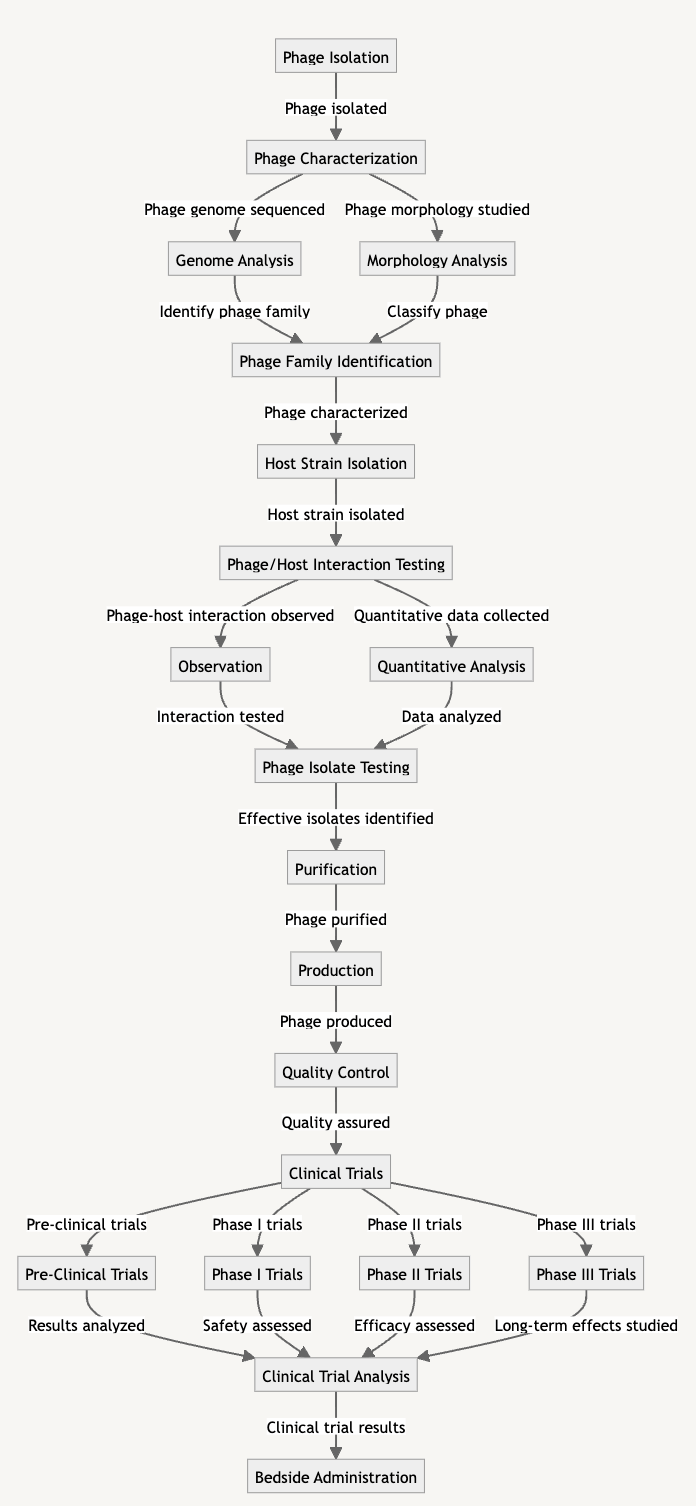
Fig 3. Mermaid graph generated by GPT-4, when asked to generate a phage therapy pipeline graph. Full prompt here.
Wrapping Up
There’s a lot of hype around AI and language models changing absolutely everything. But just like any kind of modeling, language models are only as good as the training data. It’ll reflect any biases in the training data.
I use it for a temperature check before asking Jess any questions. Most questions around phages and lab work are in the category of “obvious questions” for microbiologists, that can be easily searched for. GPT-4 is great at answering these. However, as I use GPT-4 for code, I find that its answers can fluctuate in quality. I think as one approaches mastery of a topic, GPT-4 becomes less and less useful.
I also use ChatGPT (and the OpenAI’s API) for a lot more data processing and code generation. I’m also building an entire data processing pipeline where I use code with OpenAI to extract and classify text, images, recordings, and so on.
I was going to write it out in this issue but somehow we’ve already hit the 2000 word limit, so I’ll just have to leave it as a cliff hanger for next time…
See you next time!
— Jan & Jessica
Further Reading