This week, we’ve got a great story for you — both from a phage biology perspective, and a ‘should we use AI for science?’ perspective. This is a story that was covered last month by Forbes, BBC, and several other news outlets, mainly because of how exciting it is for Google, who created a new AI tool for scientists, and for everyone who cares about AI/wonders if it’s worth the hype.
I was super excited when I saw this story (because I am always excited if we scientists get new tools to help us go faster), but also because this mainstream news-worthy story was actually involving a researcher I knew and had followed for years! It was a phage story! So I had to reach out to José and see if he’d let us interview him on Podovirus.
Excitingly, even though he’d been exhaustedly fielding media calls all month, he was happy to have a chance to talk to our community of phage scientists, to get into the nitty gritty of the science, and discuss the reality, his own skepticism, the humbling experience of working with an AI who saw something he didn’t, and what it all could mean for science. He and his colleague Tiago have published all of this, from the biology to experiment they did to test the AI tool (links below!), but first, here’s their candid conversation (and here on YouTube) — we hope you enjoy!
— Jessica
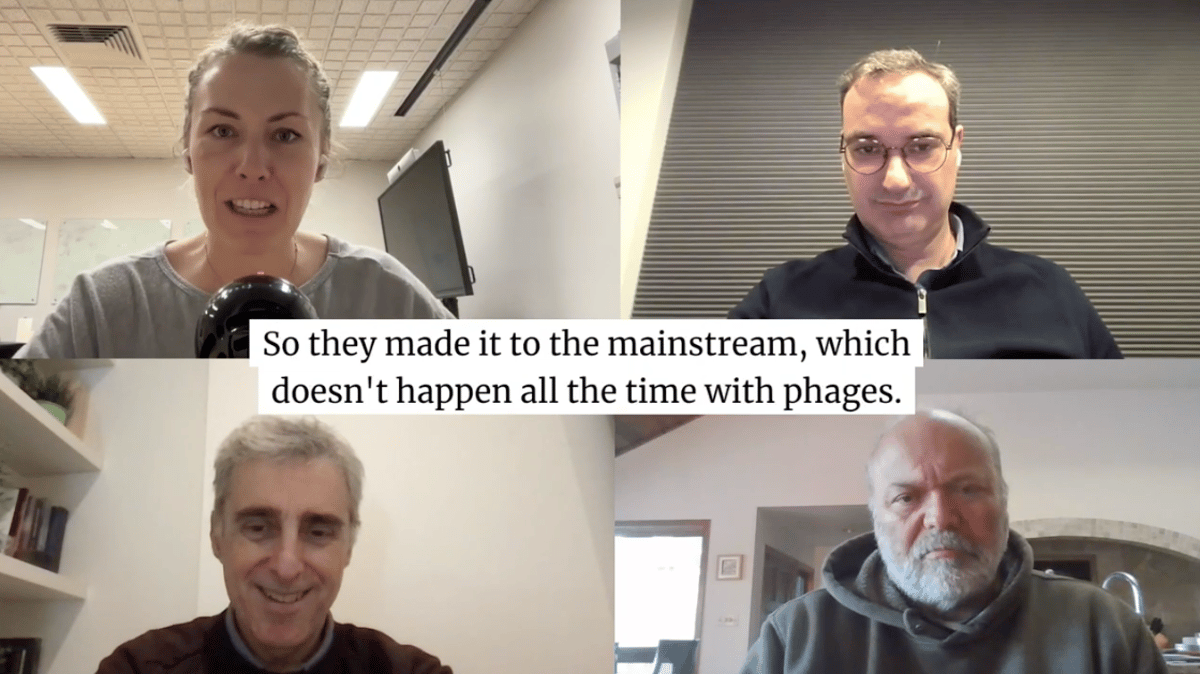
When Imperial College London professor José Penadés and his lab discovered that phage satellite entities called capsid-forming PICIs (Phage-Inducible Chromosomal Islands) could swap tails to infect different bacterial species, his lab had solved a puzzle that had eluded them for years.
Just before he was ready to publish, however, his collaborator Tiago Costa (another Imperial College Professor) told him about a new AI tool (Google’s ‘AI co-scientist’) he was testing. Tiago was really impressed with the hypotheses it was generating, and this piqued José’s interest.
The two developed a plan. Instead of waiting years to prove whether the AI was right or wrong about the experiments it was proposing for Tiago’s project, could they test the system in reverse? Could they instead first show the AI all of José’s lab’s unpublished data about PICIs, which they’d only finally figured out how to interpret, and see if it could get the same answer?
To their surprise, delight (and no doubt a bit of dismay), the AI quickly came up with the key experiments it had taken them years to consider doing.
Why? According to José and Tiago, it has to do with their own scientific biases, which made them not contemplate some ‘obvious’ explanations for the patterns they were seeing.
On the newest episode of Podovirus Podcast, Joe and I spoke with José and Tiago about the entire process — from the fascinating PICI biology question they were mystified by (and finally solved), to the experiment they did to test the AI, how it humbled and inspired them, and how they now think about how to use AI for science. Lastly, we dig into what this all means for us human scientists. What scientific biases might we each be bringing to the table? When is ‘beginner’s mind’ exactly what we need to make sense of our data? Can we use AI to help us find the patterns in our work that might be lying in plain sight?
Highlights from our conversation:
- José’s team discovered that capsid-forming phage-inducible chromosomal islands (cf-PICIs) can exist as “tailless capsids”; and that—contrary to prevailing assumptions in phage biology—these tailless cf-PICIs acquire tails after assembly and cell lysis by binding to free tails from different phages
- This modular “mix and match” capability allows PICIs to dramatically expand their host range by pairing with tails that target different bacterial species or genera
- When presented with the same question, a bunch of unpublished observations, but none of the conclusions, Google’s AI Co-Scientist tool suggested the correct mechanism (along with a set of testable hypotheses to prove it)
- The researchers estimate that using this AI tool sooner could have reduced their failed experiments (and unneeded rabbit holes) from ~90% to 50%, potentially saving years of work
- The discovery itself has significant implications for phage therapy, as it suggests a new way synthetic phages with customizable and much broader host ranges could be engineered. Finally, after years of work, they have the mechanism (and now they also have an AI tool to hopefully help them crack their next biological mystery a lot faster!)
A few snippets from the episode…
On how scientific bias delayed their discovery:
José: “We were biased. That’s the problem we had. For many years, I always thought—and all phage biology people think—that after infection, what you have are infective particles with the capsid and the tail. We didn’t understand why we had PICIs that could be induced but didn’t get transferred… We were so biased we couldn’t see what was actually happening.”
"We have been thinking a lot, okay, how this machine got the right answer. Okay. And it’s very frustrating. It’s very frustrating because we have the answer there and we didn’t see it.”
On the AI’s unbiased approach:
José: “Google [AI co-scientist] didn’t understand what’s happening here, but made the most simple connection. If you are in many different species, and to go to these species you need a tail, you are binding to different tails. That’s it… We all knew that the tail determines tropism. It was obvious, but someone needed to tell us.”
On adapters and connectors determining specificity:
Tiago: “The tropism will be always determined by the tip of the tail… But the neck region, where the port or the adapter and the connectors are, will have some role in determining that tropism… by generating a promiscuous or a very specific structure that binds only one or several phage tails.”
What’s next:
José and Tiago are now investigating whether regular phages (not just PICIs) can also exchange tails in natural environments. They’re developing IP around synthetic phage particles with customizable host ranges, which could potentially overcome one of phage therapy’s biggest limitations—narrow host specificity. Additionally, their labs are continuing to work with Google to test the co-scientist on new research questions, comparing AI-driven hypotheses with experimental results.
Listen to (or watch!) the full episode:
Learn more: